More about forecasting in cienciadedatos.net
- ARIMA and SARIMAX models with python
- Time series forecasting with machine learning
- Forecasting time series with gradient boosting: XGBoost, LightGBM and CatBoost
- Forecasting time series with XGBoost
- Global Forecasting Models: Multi-series forecasting
- Global Forecasting Models: Comparative Analysis of Single and Multi-Series Forecasting Modeling
- Probabilistic forecasting
- Forecasting with deep learning
- Forecasting energy demand with machine learning
- Forecasting web traffic with machine learning
- Intermittent demand forecasting
- Modelling time series trend with tree-based models
- Bitcoin price prediction with Python
- Stacking ensemble of machine learning models to improve forecasting
- Interpretable forecasting models
- Mitigating the Impact of Covid on Forecasting Models
- Forecasting time series with missing values
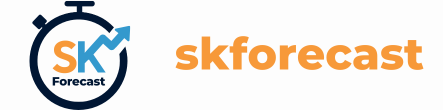
Introduction
In machine learning, stacking is an ensemble technique that combines multiple models to reduce their biases and improve predictive performance. More specifically, the predictions of each model (base models) are stacked and used as input to a final model (meta model) to compute the prediction.
Stacking is effective because it leverages the strengths of different algorithms and attempts to mitigate their individual weaknesses. By combining several models, it can capture complex patterns in the data and improve prediction accuracy.
However, stacking can be computationally expensive and requires careful tuning to avoid overfitting. To this end, it is highly recommended to train the final estimator through cross-validation. In addition, obtaining diverse and well-performing base models is critical to the success of the stacking technique.
The following example shows how to use scikit-learn and skforecast to create a forecasting model that combines several individual regressors to achieve better results.
Libraries
Libraries used in this document.
# Data processing
# ==============================================================================
import numpy as np
import pandas as pd
# Plots
# ==============================================================================
import matplotlib.pyplot as plt
import plotly.express as px
import plotly.io as pio
import plotly.offline as poff
pio.templates.default = "seaborn"
poff.init_notebook_mode(connected=True)
plt.style.use('seaborn-v0_8-darkgrid')
# Modelling and Forecasting
# ==============================================================================
from lightgbm import LGBMRegressor
from sklearn.linear_model import Ridge
from sklearn.ensemble import StackingRegressor
from sklearn.model_selection import KFold
from sklearn.preprocessing import StandardScaler
import skforecast
from skforecast.recursive import ForecasterRecursive
from skforecast.model_selection import TimeSeriesFold
from skforecast.model_selection import grid_search_forecaster
from skforecast.model_selection import backtesting_forecaster
from skforecast.datasets import fetch_dataset
# Configuration warnings
# ==============================================================================
import warnings
warnings.filterwarnings('once')
color = '\033[1m\033[38;5;208m'
print(f"{color}Version skforecast: {skforecast.__version__}")
Version skforecast: 0.16.0
Data
The data in this document represent monthly fuel consumption in Spain from 1969-01-01 to 2022-08-01. The goal is to create a model capable of forecasting the consumption over the next 12 month.
# Downloading data
# ==============================================================================
data = fetch_dataset(name = 'fuel_consumption')
data = data.loc[:"2019-01-01", ['Gasolinas']]
data = data.rename(columns = {'Gasolinas':'consumption'})
data.index.name = 'date'
data['consumption'] = data['consumption']/100000
data.head(3)
fuel_consumption ---------------- Monthly fuel consumption in Spain from 1969-01-01 to 2022-08-01. Obtained from Corporación de Reservas Estratégicas de Productos Petrolíferos and Corporación de Derecho Público tutelada por el Ministerio para la Transición Ecológica y el Reto Demográfico. https://www.cores.es/es/estadisticas Shape of the dataset: (644, 5)
consumption | |
---|---|
date | |
1969-01-01 | 1.668752 |
1969-02-01 | 1.554668 |
1969-03-01 | 1.849837 |
In addition to the past values of the series (lags), an additional variable indicating the month of the year is added. This variable is included in the model to capture the seasonality of the series.
# Calendar features
# ==============================================================================
data['month_of_year'] = data.index.month
data.head(3)
consumption | month_of_year | |
---|---|---|
date | ||
1969-01-01 | 1.668752 | 1 |
1969-02-01 | 1.554668 | 2 |
1969-03-01 | 1.849837 | 3 |
To facilitate the training of the models, the search for optimal hyperparameters, and the evaluation of their predictive accuracy, the data are divided into three separate sets: training, validation, and test.
# Split train-validation-test
# ==============================================================================
end_train = '2007-12-01 23:59:00'
end_validation = '2012-12-01 23:59:00'
data_train = data.loc[: end_train, :]
data_val = data.loc[end_train:end_validation, :]
data_test = data.loc[end_validation:, :]
print(f"Dates train : {data_train.index.min()} --- {data_train.index.max()} (n={len(data_train)})")
print(f"Dates validacion : {data_val.index.min()} --- {data_val.index.max()} (n={len(data_val)})")
print(f"Dates test : {data_test.index.min()} --- {data_test.index.max()} (n={len(data_test)})")
Dates train : 1969-01-01 00:00:00 --- 2007-12-01 00:00:00 (n=468) Dates validacion : 2008-01-01 00:00:00 --- 2012-12-01 00:00:00 (n=60) Dates test : 2013-01-01 00:00:00 --- 2019-01-01 00:00:00 (n=73)
# Interactive plot of time series
# ==============================================================================
data.loc[:end_train, 'partition'] = 'train'
data.loc[end_train:end_validation, 'partition'] = 'validation'
data.loc[end_validation:, 'partition'] = 'test'
fig = px.line(
data_frame = data.reset_index(),
x = 'date',
y = 'consumption',
color = 'partition',
title = 'Fuel consumption',
width = 700,
height = 350,
)
fig.update_layout(
width = 700,
height = 350,
margin=dict(l=20, r=20, t=35, b=20),
legend=dict(
orientation="h",
yanchor="top",
y=1,
xanchor="left",
x=0.001
)
)
fig.show()
data = data.drop(columns='partition')
Individual models
First, two individual models are trained separately - a linear regression model and a gradient boosting model - and their performance is evaluated on the test set.
LightGBM
# Create forecaster
# ==============================================================================
params_lgbm = {'learning_rate': 0.1, 'max_depth': 5, 'n_estimators': 500, 'verbose': -1}
forecaster = ForecasterRecursive(
regressor = LGBMRegressor(random_state=123, **params_lgbm),
lags = 12
)
# Backtesting on test data
# ==============================================================================
cv = TimeSeriesFold(
steps = 12,
initial_train_size = len(data.loc[:end_validation]),
fixed_train_size = False,
)
metric, predictions = backtesting_forecaster(
forecaster = forecaster,
y = data['consumption'],
exog = data['month_of_year'],
cv = cv,
metric = 'mean_squared_error'
)
metric
0%| | 0/7 [00:00<?, ?it/s]
mean_squared_error | |
---|---|
0 | 0.066196 |
Linear model
# Create forecaster
# ==============================================================================
params_ridge = {'alpha': 0.001}
forecaster = ForecasterRecursive(
regressor = Ridge(random_state=123, **params_ridge),
lags = 12,
transformer_y = StandardScaler()
)
# Backtesting on test data
# ==============================================================================
metric, predictions = backtesting_forecaster(
forecaster = forecaster,
y = data['consumption'],
exog = data['month_of_year'],
cv = cv,
metric = 'mean_squared_error'
)
metric
0%| | 0/7 [00:00<?, ?it/s]
mean_squared_error | |
---|---|
0 | 0.049092 |
StackingRegressor
With scikit-learn it is very easy to combine multiple regressors thanks to its StackingRegressor class.
The estimators
parameter corresponds to the list of the estimators (base learners) which are stacked together in parallel on the input data. It should be given as a list of names and estimators. The final_estimator
(meta model) will use the predictions of the estimators as input.
# Create stacking regressor
# ==============================================================================
estimators = [
('ridge', Ridge(random_state=123, **params_ridge)),
('lgbm', LGBMRegressor(random_state=123, **params_lgbm)),
]
stacking_regressor = StackingRegressor(
estimators = estimators,
final_estimator = Ridge(),
cv = KFold(n_splits=10, shuffle=False)
)
stacking_regressor
StackingRegressor(cv=KFold(n_splits=10, random_state=None, shuffle=False), estimators=[('ridge', Ridge(alpha=0.001, random_state=123)), ('lgbm', LGBMRegressor(max_depth=5, n_estimators=500, random_state=123, verbose=-1))], final_estimator=Ridge())In a Jupyter environment, please rerun this cell to show the HTML representation or trust the notebook.
On GitHub, the HTML representation is unable to render, please try loading this page with nbviewer.org.
StackingRegressor(cv=KFold(n_splits=10, random_state=None, shuffle=False), estimators=[('ridge', Ridge(alpha=0.001, random_state=123)), ('lgbm', LGBMRegressor(max_depth=5, n_estimators=500, random_state=123, verbose=-1))], final_estimator=Ridge())
Ridge(alpha=0.001, random_state=123)
LGBMRegressor(max_depth=5, n_estimators=500, random_state=123, verbose=-1)
Ridge()
# Create forecaster
# ==============================================================================
forecaster = ForecasterRecursive(
regressor = stacking_regressor,
lags = 12
)
# Backtesting on test data
# ==============================================================================
metric, predictions = backtesting_forecaster(
forecaster = forecaster,
y = data['consumption'],
exog = data['month_of_year'],
cv = cv,
metric = 'mean_squared_error'
)
metric
0%| | 0/7 [00:00<?, ?it/s]
mean_squared_error | |
---|---|
0 | 0.044688 |
The results obtained by stacking the two models - the linear model and the gradient boosting model - are better than the results obtained by each model separately.
Hiperparameters search
When using StackingRegressor
, the hyperparameters of individual regressors must be prefixed with the name of the regressor followed by two underlines. For example, the hyperparameter alpha
of the Ridge regressor must be specified as ridge__alpha
. The hyperparameter of the final estimator must be specified with the final_estimator__
prefix.
# Grid search of hyperparameters and lags
# ==============================================================================
param_grid = {
'ridge__alpha': [0.001, 0.01, 0.1, 1, 10],
'lgbm__n_estimators': [100, 500],
'lgbm__max_depth': [3, 5, 10],
'lgbm__learning_rate': [0.01, 0.1],
}
lags_grid = [24]
cv_Search = TimeSeriesFold(
steps = 12,
initial_train_size = len(data.loc[:end_train]),
fixed_train_size = False,
)
results_grid = grid_search_forecaster(
forecaster = forecaster,
y = data.loc[:end_validation, 'consumption'],
exog = data.loc[:end_validation, 'month_of_year'],
param_grid = param_grid,
lags_grid = lags_grid,
cv = cv_Search,
metric = 'mean_squared_error',
)
results_grid.head()
lags grid: 0%| | 0/1 [00:00<?, ?it/s]
params grid: 0%| | 0/60 [00:00<?, ?it/s]
`Forecaster` refitted using the best-found lags and parameters, and the whole data set: Lags: [ 1 2 3 4 5 6 7 8 9 10 11 12 13 14 15 16 17 18 19 20 21 22 23 24] Parameters: {'lgbm__learning_rate': 0.1, 'lgbm__max_depth': 5, 'lgbm__n_estimators': 100, 'ridge__alpha': 0.001} Backtesting metric: 0.07934431616061044
lags | lags_label | params | mean_squared_error | lgbm__learning_rate | lgbm__max_depth | lgbm__n_estimators | ridge__alpha | |
---|---|---|---|---|---|---|---|---|
0 | [1, 2, 3, 4, 5, 6, 7, 8, 9, 10, 11, 12, 13, 14... | [1, 2, 3, 4, 5, 6, 7, 8, 9, 10, 11, 12, 13, 14... | {'lgbm__learning_rate': 0.1, 'lgbm__max_depth'... | 0.079344 | 0.10 | 5.0 | 100.0 | 0.001 |
1 | [1, 2, 3, 4, 5, 6, 7, 8, 9, 10, 11, 12, 13, 14... | [1, 2, 3, 4, 5, 6, 7, 8, 9, 10, 11, 12, 13, 14... | {'lgbm__learning_rate': 0.1, 'lgbm__max_depth'... | 0.079360 | 0.10 | 5.0 | 100.0 | 0.010 |
2 | [1, 2, 3, 4, 5, 6, 7, 8, 9, 10, 11, 12, 13, 14... | [1, 2, 3, 4, 5, 6, 7, 8, 9, 10, 11, 12, 13, 14... | {'lgbm__learning_rate': 0.01, 'lgbm__max_depth... | 0.079488 | 0.01 | 3.0 | 500.0 | 0.001 |
3 | [1, 2, 3, 4, 5, 6, 7, 8, 9, 10, 11, 12, 13, 14... | [1, 2, 3, 4, 5, 6, 7, 8, 9, 10, 11, 12, 13, 14... | {'lgbm__learning_rate': 0.01, 'lgbm__max_depth... | 0.079504 | 0.01 | 3.0 | 500.0 | 0.010 |
4 | [1, 2, 3, 4, 5, 6, 7, 8, 9, 10, 11, 12, 13, 14... | [1, 2, 3, 4, 5, 6, 7, 8, 9, 10, 11, 12, 13, 14... | {'lgbm__learning_rate': 0.1, 'lgbm__max_depth'... | 0.079512 | 0.10 | 5.0 | 100.0 | 0.100 |
Once the best hyperparameters have been determined for each regressor in the ensemble, the test error is computed through back-testing.
# Backtesting the best model on test data
# ==============================================================================
metric, predictions = backtesting_forecaster(
forecaster = forecaster,
y = data['consumption'],
exog = data['month_of_year'],
cv = cv,
metric = 'mean_squared_error'
)
metric
0%| | 0/7 [00:00<?, ?it/s]
mean_squared_error | |
---|---|
0 | 0.012739 |
Feature importance
When a regressor of type StackingRegressor
is used as a regressor in a predictor, its get_feature_importances
method will not work. This is because objects of type StackingRegressor
do not have either the feature_importances
or coef_
attribute. Instead, it is necessary to inspect each of the regressors that are part of the stacking.
# Feature importances for each regressor in the stacking
# ==============================================================================
if forecaster.regressor.__class__.__name__ == 'StackingRegressor':
importancia_pred = []
for regressor in forecaster.regressor.estimators_:
try:
importancia = pd.DataFrame(
data = {
'feature': forecaster.regressor.feature_names_in_,
f'importance_{type(regressor).__name__}': regressor.coef_,
f'importance_abs_{type(regressor).__name__}': np.abs(regressor.coef_)
}
).set_index('feature')
except:
importancia = pd.DataFrame(
data = {
'feature': forecaster.regressor.feature_names_in_,
f'importance_{type(regressor).__name__}': regressor.feature_importances_,
f'importance_abs_{type(regressor).__name__}': np.abs(regressor.feature_importances_)
}
).set_index('feature')
importancia_pred.append(importancia)
importancia_pred = pd.concat(importancia_pred, axis=1)
else:
importancia_pred = forecaster.get_feature_importances()
importancia_pred['importance_abs'] = importancia_pred['importance'].abs()
importancia_pred = importancia_pred.sort_values(by='importance_abs', ascending=False)
importancia_pred.head(5)
importance_Ridge | importance_abs_Ridge | importance_LGBMRegressor | importance_abs_LGBMRegressor | |
---|---|---|---|---|
feature | ||||
lag_1 | 0.016128 | 0.016128 | 58 | 58 |
lag_2 | 0.226245 | 0.226245 | 63 | 63 |
lag_3 | 0.185437 | 0.185437 | 44 | 44 |
lag_4 | 0.206009 | 0.206009 | 54 | 54 |
lag_5 | 0.105056 | 0.105056 | 38 | 38 |
Session information
import session_info
session_info.show(html=False)
----- lightgbm 4.6.0 matplotlib 3.10.1 numpy 1.26.4 pandas 2.2.3 plotly 6.0.1 session_info v1.0.1 skforecast 0.16.0 sklearn 1.6.1 ----- IPython 9.1.0 jupyter_client 8.6.3 jupyter_core 5.7.2 notebook 6.5.7 ----- Python 3.12.9 | packaged by Anaconda, Inc. | (main, Feb 6 2025, 18:56:27) [GCC 11.2.0] Linux-6.11.0-25-generic-x86_64-with-glibc2.39 ----- Session information updated at 2025-05-13 22:59
Citation
How to cite this document
If you use this document or any part of it, please acknowledge the source, thank you!
Stacking ensemble of machine learning models to improve forecasting by Joaquín Amat Rodrigo and Javier Escobar Ortiz, available under Attribution-NonCommercial-ShareAlike 4.0 International (CC BY-NC-SA 4.0 DEED) at https://www.cienciadedatos.net/documentos/py52-stacking-ensemble-models-forecasting.html
How to cite skforecast
If you use skforecast for a publication, we would appreciate it if you cite the published software.
Zenodo:
Amat Rodrigo, Joaquin, & Escobar Ortiz, Javier. (2024). skforecast (v0.16.0). Zenodo. https://doi.org/10.5281/zenodo.8382788
APA:
Amat Rodrigo, J., & Escobar Ortiz, J. (2024). skforecast (Version 0.16.0) [Computer software]. https://doi.org/10.5281/zenodo.8382788
BibTeX:
@software{skforecast, author = {Amat Rodrigo, Joaquin and Escobar Ortiz, Javier}, title = {skforecast}, version = {0.16.0}, month = {05}, year = {2025}, license = {BSD-3-Clause}, url = {https://skforecast.org/}, doi = {10.5281/zenodo.8382788} }
Did you like the article? Your support is important
Your contribution will help me to continue generating free educational content. Many thanks! 😊
This work by Joaquín Amat Rodrigo and Javier Escobar Ortiz is licensed under a Attribution-NonCommercial-ShareAlike 4.0 International.
Allowed:
-
Share: copy and redistribute the material in any medium or format.
-
Adapt: remix, transform, and build upon the material.
Under the following terms:
-
Attribution: You must give appropriate credit, provide a link to the license, and indicate if changes were made. You may do so in any reasonable manner, but not in any way that suggests the licensor endorses you or your use.
-
NonCommercial: You may not use the material for commercial purposes.
-
ShareAlike: If you remix, transform, or build upon the material, you must distribute your contributions under the same license as the original.