More about forecasting in cienciadedatos.net
- ARIMA and SARIMAX models with python
- Time series forecasting with machine learning
- Forecasting time series with gradient boosting: XGBoost, LightGBM and CatBoost
- Forecasting time series with XGBoost
- Global Forecasting Models: Multi-series forecasting
- Global Forecasting Models: Comparative Analysis of Single and Multi-Series Forecasting Modeling
- Probabilistic forecasting
- Forecasting with deep learning
- Forecasting energy demand with machine learning
- Forecasting web traffic with machine learning
- Intermittent demand forecasting
- Modelling time series trend with tree-based models
- Bitcoin price prediction with Python
- Stacking ensemble of machine learning models to improve forecasting
- Interpretable forecasting models
- Mitigating the Impact of Covid on Forecasting Models
- Forecasting time series with missing values
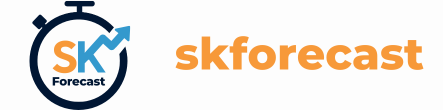
Introduction
Anomaly detection involves the identification of data points, patterns or events that deviate significantly from expected behaviour. In the context of time series data, these anomalies, often referred to as outliers, can arise from various sources such as changes in the underlying data generating process, measurement errors or unexpected external events. Detecting anomalies in time series is particularly challenging due to the inherent characteristics of these data, including time dependencies, trends, seasonality and noise. These factors can obscure the distinction between true anomalies and normal variation.
Despite these challenges, anomaly detection in time series is critical. Undetected anomalies can significantly affect the performance of forecasting models by biasing their predictions and compromising their accuracy. Therefore, identifying and carefully analysing anomalies is essential to maintaining model reliability and improving the quality of insights derived from the data.
This article explores a specific approach to anomaly detection in time series: measuring the residuals of a forecasting model. Residuals represent the differences between observed values and the corresponding model predictions, and provide a valuable perspective for identifying anomalies that the model is struggling to accurately predict.
✎ Note
There are numerous approaches to anomaly detection in time series, including statistical methods, seasonal-trend decomposition, machine learning techniques like classification and clustering, as well as autoencoders, among others. Each method comes with its own strengths and limitations, and the choice of approach should align with the specific characteristics of the data and the objectives of the analysis.Libraries
The libraries used in this document are:
# Data processing
# ==============================================================================
import numpy as np
import pandas as pd
from skforecast.datasets import fetch_dataset
# Plots
# ==============================================================================
import matplotlib.pyplot as plt
from skforecast.plot import set_dark_theme
# Modelling and Forecasting
# ==============================================================================
import skforecast
import sklearn
from sklearn.ensemble import HistGradientBoostingRegressor
from sklearn.preprocessing import StandardScaler
from sklearn.pipeline import make_pipeline
from feature_engine.datetime import DatetimeFeatures
from feature_engine.creation import CyclicalFeatures
from skforecast.recursive import ForecasterRecursive
from skforecast.model_selection import TimeSeriesFold
from skforecast.model_selection import backtesting_forecaster
from skforecast.preprocessing import RollingFeatures
# Warnings configuration
# ==============================================================================
import warnings
warnings.filterwarnings('once')
print('Version skforecast:', skforecast.__version__)
print('Version sklearn:', sklearn.__version__)
Version skforecast: 0.16.0 Version sklearn: 1.6.1
Data
# Download data
# ==============================================================================
data = fetch_dataset("ett_m1")
print(f"Data shape: {data.shape}")
data.head(3)
ett_m1 ------ Data from an electricity transformer station was collected between July 2016 and July 2018 (2 years x 365 days x 24 hours x 4 intervals per hour = 70,080 data points). Each data point consists of 8 features, including the date of the point, the predictive value "Oil Temperature (OT)", and 6 different types of external power load features: High UseFul Load (HUFL), High UseLess Load (HULL), Middle UseFul Load (MUFL), Middle UseLess Load (MULL), Low UseFul Load (LUFL), Low UseLess Load (LULL). Zhou, Haoyi & Zhang, Shanghang & Peng, Jieqi & Zhang, Shuai & Li, Jianxin & Xiong, Hui & Zhang, Wancai. (2020). Informer: Beyond Efficient Transformer for Long Sequence Time-Series Forecasting. [10.48550/arXiv.2012.07436](https://arxiv.org/abs/2012.07436). https://github.com/zhouhaoyi/ETDataset Shape of the dataset: (69680, 7) Data shape: (69680, 7)
HUFL | HULL | MUFL | MULL | LUFL | LULL | OT | |
---|---|---|---|---|---|---|---|
date | |||||||
2016-07-01 00:00:00 | 5.827 | 2.009 | 1.599 | 0.462 | 4.203 | 1.340 | 30.531000 |
2016-07-01 00:15:00 | 5.760 | 2.076 | 1.492 | 0.426 | 4.264 | 1.401 | 30.459999 |
2016-07-01 00:30:00 | 5.760 | 1.942 | 1.492 | 0.391 | 4.234 | 1.310 | 30.038000 |
# Select data
# ==============================================================================
data = data.loc["20170101":, ["OT"]]
Few synthetic anomalies are added to the time series data to illustrate the detection process.
# Synthetic anomalies
# ==============================================================================
rng = np.random.default_rng(4631789)
n_anomalies = 20
anomalies_loc = data.index[rng.uniform(low=0, high=len(data), size=n_anomalies).astype(int)]
data.loc[anomalies_loc, "OT"] = data.loc[anomalies_loc, "OT"] * rng.choice([0.5, 2], size=n_anomalies)
# Plot data
# ==============================================================================
set_dark_theme()
fig, ax = plt.subplots(figsize=(7, 3))
ax.plot(data["OT"], linewidth=1)
ax.scatter(anomalies_loc, data.loc[anomalies_loc, "OT"], color='orange', label='Anomalies', zorder=2)
ax.set_title('OT')
ax.legend();
# Data partition: train-test
# ==============================================================================
end_train = '2018-04-03 23:59:00'
data_train = data.loc[: end_train, :]
data_test = data.loc[end_train:, :]
anomalies_loc_train = [date for date in anomalies_loc if date <= pd.to_datetime(end_train)]
anomalies_loc_test = [date for date in anomalies_loc if date > pd.to_datetime(end_train)]
print(f"Dates train : {data_train.index.min()} --- {data_train.index.max()} (n={len(data_train)})")
print(f" Anomalies train: {len(anomalies_loc_train)}")
print(f"Dates test : {data_test.index.min()} --- {data_test.index.max()} (n={len(data_test)})")
print(f" Anomalies test : {len(anomalies_loc_test)}")
Dates train : 2017-01-01 00:00:00 --- 2018-04-03 23:45:00 (n=43968) Anomalies train: 16 Dates test : 2018-04-04 00:00:00 --- 2018-06-26 19:45:00 (n=8048) Anomalies test : 4
# Plot partitions
# ==============================================================================
fig, ax = plt.subplots(figsize=(7, 3))
ax.plot(data_train['OT'], label='Train', linewidth=1)
ax.plot(data_test['OT'], label='Test', linewidth=1)
ax.scatter(anomalies_loc, data.loc[anomalies_loc, "OT"], color='orange', label='Anomalies', zorder=3)
ax.set_title('OT')
ax.legend();
Feature engineering
Additional exogenous features are created based on:
Lagged values of the exogenous series.
Rolling statistics of the exogenous series.
Calendar features.
# Calendar features
# ==============================================================================
features_to_extract = [
'year',
'month',
'week',
'day_of_week',
'hour'
]
calendar_transformer = DatetimeFeatures(
variables = 'index',
features_to_extract = features_to_extract,
drop_original = False,
)
# Cliclical encoding of calendar features
# ==============================================================================
features_to_encode = [
"month",
"week",
"day_of_week",
"hour",
]
max_values = {
"month": 12,
"week": 52,
"day_of_week": 7,
"hour": 24,
}
cyclical_encoder = CyclicalFeatures(
variables = features_to_encode,
max_values = max_values,
drop_original = True
)
exog_transformer = make_pipeline(
calendar_transformer,
cyclical_encoder
)
display(exog_transformer)
data = exog_transformer.fit_transform(data)
# Remove rows with NaNs created by lag features
data = data.dropna()
exog_features = data.columns.difference(['OT']).tolist()
display(data.head(3))
Pipeline(steps=[('datetimefeatures', DatetimeFeatures(drop_original=False, features_to_extract=['year', 'month', 'week', 'day_of_week', 'hour'], variables='index')), ('cyclicalfeatures', CyclicalFeatures(drop_original=True, max_values={'day_of_week': 7, 'hour': 24, 'month': 12, 'week': 52}, variables=['month', 'week', 'day_of_week', 'hour']))])In a Jupyter environment, please rerun this cell to show the HTML representation or trust the notebook.
On GitHub, the HTML representation is unable to render, please try loading this page with nbviewer.org.
Pipeline(steps=[('datetimefeatures', DatetimeFeatures(drop_original=False, features_to_extract=['year', 'month', 'week', 'day_of_week', 'hour'], variables='index')), ('cyclicalfeatures', CyclicalFeatures(drop_original=True, max_values={'day_of_week': 7, 'hour': 24, 'month': 12, 'week': 52}, variables=['month', 'week', 'day_of_week', 'hour']))])
DatetimeFeatures(drop_original=False, features_to_extract=['year', 'month', 'week', 'day_of_week', 'hour'], variables='index')
CyclicalFeatures(drop_original=True, max_values={'day_of_week': 7, 'hour': 24, 'month': 12, 'week': 52}, variables=['month', 'week', 'day_of_week', 'hour'])
OT | year | month_sin | month_cos | week_sin | week_cos | day_of_week_sin | day_of_week_cos | hour_sin | hour_cos | |
---|---|---|---|---|---|---|---|---|---|---|
date | ||||||||||
2017-01-01 00:00:00 | 10.200 | 2017 | 0.5 | 0.866025 | -2.449294e-16 | 1.0 | -0.781831 | 0.62349 | 0.0 | 1.0 |
2017-01-01 00:15:00 | 9.989 | 2017 | 0.5 | 0.866025 | -2.449294e-16 | 1.0 | -0.781831 | 0.62349 | 0.0 | 1.0 |
2017-01-01 00:30:00 | 10.130 | 2017 | 0.5 | 0.866025 | -2.449294e-16 | 1.0 | -0.781831 | 0.62349 | 0.0 | 1.0 |
Train model
A forecaster is trained to allow its predictions to be compared with observed values. The more effectively the model learns the underlying patterns in the data, the more accurate its predictions become. This improved accuracy enhances confidence that deviations between observed and predicted values represent genuine anomalies.
# Create a forecaster
# ==============================================================================
window_features = RollingFeatures(stats=['mean', 'min', 'max'], window_sizes=24)
forecaster = ForecasterRecursive(
regressor = HistGradientBoostingRegressor(random_state=15926, loss='quantile', quantile=0.5),
lags = [1, 3, 4, 5, 6, 13, 14, 15, 16, 17],
differentiation = 1,
transformer_y = StandardScaler(),
window_features = window_features,
)
forecaster.fit(
y = data.loc[:end_train, 'OT'],
exog = data.loc[:end_train, exog_features]
)
forecaster
ForecasterRecursive
General Information
- Regressor: HistGradientBoostingRegressor
- Lags: [ 1 3 4 5 6 13 14 15 16 17]
- Window features: ['roll_mean_24', 'roll_min_24', 'roll_max_24']
- Window size: 25
- Series name: OT
- Exogenous included: True
- Weight function included: False
- Differentiation order: 1
- Creation date: 2025-05-17 21:00:52
- Last fit date: 2025-05-17 21:00:53
- Skforecast version: 0.16.0
- Python version: 3.12.9
- Forecaster id: None
Exogenous Variables
-
day_of_week_cos, day_of_week_sin, hour_cos, hour_sin, month_cos, month_sin, week_cos, week_sin, year
Data Transformations
- Transformer for y: StandardScaler()
- Transformer for exog: None
Training Information
- Training range: [Timestamp('2017-01-01 00:00:00'), Timestamp('2018-04-03 23:45:00')]
- Training index type: DatetimeIndex
- Training index frequency: 15min
Regressor Parameters
-
{'categorical_features': 'from_dtype', 'early_stopping': 'auto', 'interaction_cst': None, 'l2_regularization': 0.0, 'learning_rate': 0.1, 'loss': 'quantile', 'max_bins': 255, 'max_depth': None, 'max_features': 1.0, 'max_iter': 100, 'max_leaf_nodes': 31, 'min_samples_leaf': 20, 'monotonic_cst': None, 'n_iter_no_change': 10, 'quantile': 0.5, 'random_state': 15926, 'scoring': 'loss', 'tol': 1e-07, 'validation_fraction': 0.1, 'verbose': 0, 'warm_start': False}
Fit Kwargs
-
{}
Anomaly detection
After the model is trained, predictions are generated for both the training set and the test set. This is achieved through a backtesting process. For this example it is assumed that 24 steps ahead are predicted in each iteration.
# Backtesting on training data
# ==============================================================================
cv = TimeSeriesFold(
initial_train_size = None, # This will use all data, assuming that the model has been trained
steps = 24, # All hours of next day
differentiation = 1,
)
metric_train, predictions_train = backtesting_forecaster(
forecaster = forecaster,
y = data.loc[:end_train, 'OT'],
exog = data.loc[:end_train, exog_features],
cv = cv,
metric = 'mean_absolute_error',
n_jobs = 'auto',
verbose = False,
show_progress = True
)
results_train = predictions_train.copy()
results_train['y_true'] = data.loc[:end_train, 'OT']
results_train['residual'] = results_train['y_true'] - results_train['pred']
results_train['residual_abs'] = np.abs(results_train['residual'])
results_train.head(3)
0%| | 0/1831 [00:00<?, ?it/s]
pred | y_true | residual | residual_abs | |
---|---|---|---|---|
2017-01-01 06:15:00 | 10.101177 | 10.833 | 0.731823 | 0.731823 |
2017-01-01 06:30:00 | 10.094863 | 11.537 | 1.442136 | 1.442136 |
2017-01-01 06:45:00 | 10.089609 | 11.537 | 1.447390 | 1.447390 |
In the training set, there are a total of 16 anomalies. Next, the 16 largest residuals are identified, and the corresponding time points are extracted. These time points are then compared with the actual anomalies to determine the model's ability to detect them.
# Plot predictions vs real value and residuals on training data
# ==============================================================================
fig, axs = plt.subplots(
2, 1, figsize=(7, 5), gridspec_kw={"height_ratios": [2, 1]}, sharex=True
)
axs[0].plot(data.loc[:end_train, "OT"], label="Real value")
axs[0].plot(predictions_train["pred"], label="Prediction")
axs[0].scatter(
anomalies_loc_train,
data.loc[anomalies_loc_train, "OT"],
zorder=2,
color="orange",
label="Anomalies",
)
axs[0].set_title("Backtesting on train data")
axs[0].legend()
axs[1].plot(results_train["residual_abs"], label="Absolute residuals", zorder=2)
axs[1].set_title("Absolute residuals")
top_residuals_train = (
results_train.sort_values("residual_abs", ascending=False).head(len(anomalies_loc_train))
)
top_residuals_train["is_anomaly"] = top_residuals_train.index.isin(anomalies_loc_train)
axs[1].scatter(
anomalies_loc_train,
results_train.loc[anomalies_loc_train, "residual_abs"],
zorder=2,
color="orange",
label="Anomalies",
)
plt.tight_layout()
display(top_residuals_train)
top_residuals_train["is_anomaly"].value_counts()
pred | y_true | residual | residual_abs | is_anomaly | |
---|---|---|---|---|---|
2017-06-21 19:15:00 | 19.676958 | 38.549999 | 18.873041 | 18.873041 | True |
2017-08-23 03:00:00 | 15.963411 | 32.360001 | 16.396589 | 16.396589 | True |
2017-04-07 21:00:00 | 13.038298 | 28.420000 | 15.381702 | 15.381702 | True |
2017-06-05 00:45:00 | 14.800458 | 28.982000 | 14.181542 | 14.181542 | True |
2017-03-04 17:15:00 | 13.874956 | 26.872000 | 12.997044 | 12.997044 | True |
2017-06-01 22:30:00 | 22.993038 | 10.482000 | -12.511038 | 12.511038 | False |
2017-03-05 20:00:00 | 12.504316 | 24.340000 | 11.835685 | 11.835685 | True |
2017-06-01 22:45:00 | 22.969251 | 11.396000 | -11.573251 | 11.573251 | False |
2017-05-12 04:30:00 | 17.299185 | 7.105000 | -10.194185 | 10.194185 | False |
2017-07-13 22:45:00 | 21.299531 | 11.115000 | -10.184532 | 10.184532 | True |
2018-03-15 21:00:00 | 11.082166 | 0.985000 | -10.097166 | 10.097166 | False |
2017-11-10 02:45:00 | 8.333463 | 18.430000 | 10.096537 | 10.096537 | True |
2017-04-17 04:30:00 | 17.505625 | 7.457000 | -10.048625 | 10.048625 | False |
2018-03-15 21:15:00 | 11.061701 | 1.126000 | -9.935701 | 9.935701 | False |
2018-03-15 20:45:00 | 11.108253 | 1.196000 | -9.912253 | 9.912253 | False |
2017-05-11 17:15:00 | 23.697282 | 13.788000 | -9.909282 | 9.909282 | False |
is_anomaly True 8 False 8 Name: count, dtype: int64
print(f"Percentage of true positives: {100 * top_residuals_train['is_anomaly'].mean():.2f}%")
print(f"Percentage of false positives: {100 * (~top_residuals_train['is_anomaly']).mean():.2f}%")
Percentage of true positives: 50.00% Percentage of false positives: 50.00%
Same process is repeated for the test set, where there are a total of 4 anomalies.
# Backtesting on test
# ==============================================================================
cv = TimeSeriesFold(
initial_train_size = len(data_train),
steps = 24, # all hours of next day
refit = False,
differentiation = 1,
)
metric_test, predictions_test = backtesting_forecaster(
forecaster = forecaster,
y = data.loc[:, 'OT'],
exog = data.loc[:, exog_features],
cv = cv,
metric = 'mean_absolute_error',
n_jobs = 'auto',
verbose = False,
show_progress = True
)
results_test = predictions_test.copy()
results_test['y_true'] = data.loc[end_train:, 'OT']
results_test['residual'] = results_test['y_true'] - results_test['pred']
results_test['residual_abs'] = np.abs(results_test['residual'])
results_test.head(3)
0%| | 0/336 [00:00<?, ?it/s]
pred | y_true | residual | residual_abs | |
---|---|---|---|---|
2018-04-04 00:00:00 | 10.539706 | 9.919 | -0.620706 | 0.620706 |
2018-04-04 00:15:00 | 10.515761 | 9.004 | -1.511761 | 1.511761 |
2018-04-04 00:30:00 | 10.487109 | 8.723 | -1.764109 | 1.764109 |
# Plot predictions vs real value and residuals on test data
# ==============================================================================
fig, axs = plt.subplots(
2, 1, figsize=(8, 5), gridspec_kw={"height_ratios": [2, 1]}, sharex=True
)
axs[0].plot(data.loc[end_train:, "OT"], label="Real value")
axs[0].plot(results_test["pred"], label="Prediction")
axs[0].scatter(
anomalies_loc_test,
data.loc[anomalies_loc_test, "OT"],
color="orange",
zorder=2,
label="Anomalies",
)
axs[0].set_title("Backtesting on test data")
axs[0].legend()
axs[1].plot(results_test["residual_abs"], label="Absolute residuals")
axs[1].set_title("Absolute residuals")
axs[1].scatter(
anomalies_loc_test,
results_test.loc[anomalies_loc_test, "residual_abs"],
color="orange",
zorder=2,
label="Anomalies",
)
plt.tight_layout()
top_residuals_test = results_test.sort_values('residual_abs', ascending=False).head(len(anomalies_loc_test))
top_residuals_test['is_anomaly'] = top_residuals_test.index.isin(anomalies_loc_test)
display(top_residuals_test)
top_residuals_test['is_anomaly'].value_counts()
pred | y_true | residual | residual_abs | is_anomaly | |
---|---|---|---|---|---|
2018-05-07 20:45:00 | 6.913693 | 17.728001 | 10.814307 | 10.814307 | True |
2018-05-18 16:15:00 | 14.043144 | 5.557000 | -8.486144 | 8.486144 | False |
2018-05-18 16:30:00 | 14.080936 | 6.402000 | -7.678936 | 7.678936 | False |
2018-05-26 02:15:00 | 7.083487 | 14.632000 | 7.548513 | 7.548513 | True |
is_anomaly True 2 False 2 Name: count, dtype: int64
In both the training and test sets, the observations with the largest residuals are true anomalies. However, when selecting as many top residuals as there are anomalies in the data, only 50% of these are true anomalies, the rest being false positives.
This approach has a significant limitation: anomalies in the data can distort predictions if they are included in the lags used by the model. This often results in as many false positives as true anomalies, as the accuracy of the model is compromised by the anomalies themselves. One way to mitigate this problem is to iteratively remove/replace the detected anomalies from the data and retrain the model. This process can be repeated until the model no longer detects anomalies, at which point the remaining anomalies are likely to be real.
⚠ Warning
Removing anomalies should be done with caution. Since they have been identified because the model is unable to predict them accurately, removing them is likely to improve the model's performance. However, if the anomalies are not due to errors or measurement problems, removing them could result in the loss of valuable information. Furthermore, if similar anomalies are expected to occur in the future, the model will not be able to learn from them and its performance will be compromised.Session information
import session_info
session_info.show(html=False)
----- feature_engine 1.8.3 matplotlib 3.10.1 numpy 2.2.5 pandas 2.2.3 session_info v1.0.1 skforecast 0.16.0 sklearn 1.6.1 ----- IPython 9.1.0 jupyter_client 8.6.3 jupyter_core 5.7.2 notebook 6.5.7 ----- Python 3.12.9 | packaged by Anaconda, Inc. | (main, Feb 6 2025, 18:56:27) [GCC 11.2.0] Linux-6.11.0-25-generic-x86_64-with-glibc2.39 ----- Session information updated at 2025-05-17 21:01
Citation
How to cite this document
If you use this document or any part of it, please acknowledge the source, thank you!
Time series anomaly detection by Joaquín Amat Rodrigo and Javier Escobar Ortiz, available under Attribution-NonCommercial-ShareAlike 4.0 International (CC BY-NC-SA 4.0 DEED) at https://cienciadedatos.net/documentos/py62-time-series-anomaly-detection.html
How to cite skforecast
If you use skforecast for a publication, we would appreciate it if you cite the published software.
Zenodo:
Amat Rodrigo, Joaquin, & Escobar Ortiz, Javier. (2025). skforecast (v0.16.0). Zenodo. https://doi.org/10.5281/zenodo.8382788
APA:
Amat Rodrigo, J., & Escobar Ortiz, J. (2025). skforecast (Version 0.16.0) [Computer software]. https://doi.org/10.5281/zenodo.8382788
BibTeX:
@software{skforecast, author = {Amat Rodrigo, Joaquin and Escobar Ortiz, Javier}, title = {skforecast}, version = {0.16.0}, month = {05}, year = {2025}, license = {BSD-3-Clause}, url = {https://skforecast.org/}, doi = {10.5281/zenodo.8382788} }
Did you like the article? Your support is important
Your contribution will help me to continue generating free educational content. Many thanks! 😊
This work by Joaquín Amat Rodrigo and Javier Escobar Ortiz is licensed under a Attribution-NonCommercial-ShareAlike 4.0 International.
Allowed:
-
Share: copy and redistribute the material in any medium or format.
-
Adapt: remix, transform, and build upon the material.
Under the following terms:
-
Attribution: You must give appropriate credit, provide a link to the license, and indicate if changes were made. You may do so in any reasonable manner, but not in any way that suggests the licensor endorses you or your use.
-
NonCommercial: You may not use the material for commercial purposes.
-
ShareAlike: If you remix, transform, or build upon the material, you must distribute your contributions under the same license as the original.